An Entirely New Approach to Drug Discovery for AD
UCSF BCHSI data scientist’s research draws attention to an entirely new approach to drug discovery for Alzheimer’s.
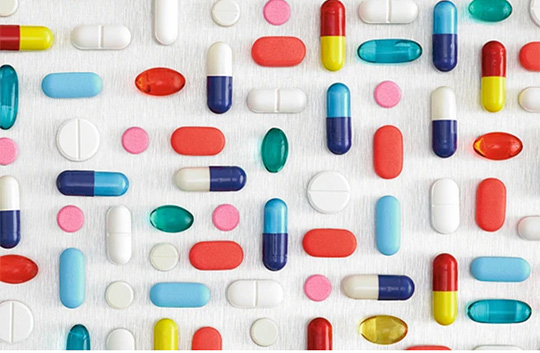
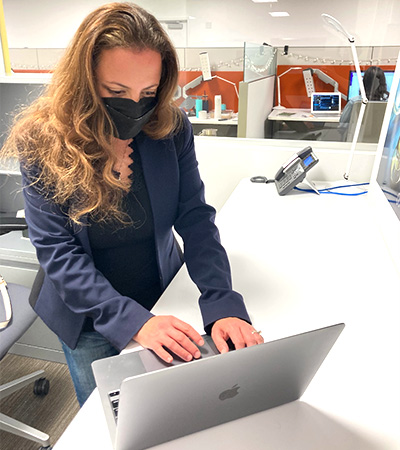
Photo credit: Nature Aging, October 2021.
Marina Sirota, PhD, an Associate Professor of the Bakar Computational Health Sciences Institute (BCHSI), is co-senior author of a new study, “Experimental and real-world evidence supporting the computational repurposing of bumetanide for APOE4-related Alzheimer’s disease,” which was recently published as the cover story of Nature Aging.
Her research gets us one step closer to preventing, diagnosing and potentially treating Alzheimer’s disease. In this interview, Dr. Sirota describes key results and provides context regarding the evolution of the field of data science.
What was the most important finding?
The most important finding was computationally identifying bumetanide, an existing FDA-approved drug, as a potential therapeutic for Alzheimer’s disease. The prediction was validated with extensive animal and human cell studies and even further validated by leveraging real-world clinical data across two independent health systems. In this manner, our work was bookended by data science.
Together with my close collaborator, the Gladstone Senior Investigator Yadong Huang, MD, PhD, who was a co-senior author of the study, we have filed a patent on this work and aim to next move forward with clinical trials. Because bumetanide is already FDA approved for other indications, it can move quickly into clinical trials, which I estimate would halve the typical drug development timeline.
What else is noteworthy about this study?
- By focusing on identifying therapeutics for patients with the APOE4/4 genotype, this study looks at Alzheimer’s disease through the lens of precision medicine - an approach that seeks to understand those who are at highest risk based not only on lifestyle and environmental factors, but also on their genetic makeup.
- Using this new approach, we confirmed our understanding that Alzheimer’s disease is not a single disease. Instead, we demonstrate there are sub-groups of patients that might respond differently to different treatments.
Editorial Note: According to STAT News coverage of Sirota and Huang’s work, the team provided “a surprising twist that suggests scientists have a lot more to learn about the root cause of the neurodegenerative condition.” Their work indicates that there are many cellular and molecular changes in Alzheimer’s disease patients besides amyloid and tau plaques. - It demonstrates the value of using real-world evidence (i.e., existing patient and drug data sets), alongside traditional scientific approaches (i.e., animal models and experimentation on human brains). Because an existing drug was identified as a potential therapeutic, it can move quickly to clinical trials.
- Finally, this study is an example of a successful collaboration between computational scientists, wet-lab neurobiologists, and clinical experts showing how diverse and complementary scientific teamwork can successfully guide discovery efforts.
What do you find most critical about your work today?
My work addresses outcomes across the lifespan, ranging from pregnancy to Alzheimer’s disease, using data science. I enjoy working on issues that support some of our most vulnerable populations. Thanks to the radical change in data science and the opportunities to develop solutions in completely new ways, we have two amazing opportunities:
- Educating researchers about the public and other types of data that are now available, as many are unaware that, for the majority of all funded studies, the data generated must be made publicly available. In addition, clinical data is a remarkable resource that can be leveraged in translational research. In the case of this study, we used a publicly available gene expression database with 215 disease samples and 1,500 drug profiles to make the initial prediction.
- Training the next generation of researchers who use the data to understand the difference between signal and noise, and to know which computational approaches can be applied to answer biomedical questions.
Training Scientists
As a result of the growing gap between the new tools and those who can use them well, I dedicate a good deal of time to training the next generation of biomedical data scientists. Together with Tomiko Oskotsky, MD I run a high school program at UCSF called “AI4ALL.” Each year, we accept about 30 high school students, primarily girls and young adults from underrepresented minorities, to learn ways of applying machine learning to biomedical questions. It is fantastic to see what these kids can learn in only three weeks. We developed a curriculum which included an introduction to statistics and machine learning, Python programming, faculty guest lecturers, personal growth sessions, and research projects. The program concludes with a symposium where the students present their work. These high school students and the new generation of trainees have the skill sets we need to advance the field of precision medicine.
What drew you to become a healthcare data scientist?
My family has had the biggest influence on me in terms of my decision to pursue academic science as a career. My parents encouraged my interest in math and the sciences and have supported me tremendously at all stages of my career. My father, Vladimir Sirota, is an aeronautical engineer, so my interest in math and computation started when he would solve math problems with me as a child. My mom, Galina Rozina, has had a long and successful academic career both in Russia as an architect and in the U.S., diving further into computer arts, 3D animation, and visual effects. She has been an amazing role model and has always encouraged me to pursue a similar path.
My father was diagnosed with multiple sclerosis (MS), shortly after I was born. It is a devastating and debilitating disease that has affected his everyday life and ability to work. My family moved to San Francisco from Russia and a big reason for that was for my father to get the best possible care at the multiple sclerosis center at UCSF. Seeing my dad battle this disease for the past 37 years has further shaped my passion for diagnostic and therapeutic discovery in order to better understand the role of the immune system in disease.
My interest in applying data science to biomedicine also stems from my experience attending a local public high school, Abraham Lincoln High, in the Sunset district of San Francisco, and I actually grew up living very close to the UCSF Parnassus campus! Because I enjoyed math, I was interested in studying computer science. At the same time, in my high school biotechnology class, I had the opportunity to learn more about genetics and wanted to find an intersection between the two fields that I was so interested in.
I found this intersection as an undergraduate at Stanford. I was drawn immediately to the field of biomedical informatics, and have been fortunate to have had incredible mentors along the way, including Serafim Batzoglou (my undergraduate research advisor), David Cox (whom I worked with at Pfizer), and, of course, Atul Butte, the Director of UCSF Bakar Computational Health Sciences Institute (BCHSI).
Atul hired me as his first graduate student when he was starting his academic career at Stanford in 2006. He introduced me to the field of translational bioinformatics. I pivoted from basic science applications to translational medicine, and began working on developing computational methods to impact diagnostics and therapeutics, which I have been pursuing for the last 15 years.
Please describe your career and where you are headed next.
My career has followed an unusual arc in that I returned to academia after having spent time in industry, working at Pfizer, where I was developing precision medicine strategies in drug discovery. After Pfizer, I came to UCSF to join Atul when he moved from Stanford to UCSF in 2015. I was thrilled to be a part of the creation of something completely new - the UCSF Bakar Computational Health Sciences Institute (BCHSI), and started my own lab here, the Sirota Lab.
I am passionate about applying computational approaches to study a number of disease areas across the lifespan. My interest in the role of the immune system in disease stems from my family’s personal experience. In addition to our work on Alzheimer’s disease, my team studies autoimmunity--diseases such as MS, lupus, rheumatoid arthritis and others. We are also dedicated to women’s health including adverse pregnancy outcomes such as preterm birth and recurrent pregnancy loss. My team is funded by the March of Dimes to develop an open data repository for prematurity research. In collaboration with Ruth Lathi and Michael Snyder at Stanford, and Aleksandar Rajkovic at UCSF, we recently launched a new program to study the genetics of recurrent pregnancy loss. I collaborate very closely with Linda Guidice, leveraging multi-omics data in a precision medicine approach to endometriosis, a painful condition which affects 10% of all women and which often results in infertility and other adverse outcomes. We have recently launched the UCSF Stanford Endometriosis Center for Discovery, Innovation, Training and Community Engagement (ENACT) in collaboration with her and Brice Gaudilliere at Stanford.
About Marina Sirota
Marina Sirota is currently an associate professor at the Bakar Computational Health Sciences Institute (BCHSI) at UCSF. Prior to that, she worked as a senior research scientist at Pfizer where she focused on developing precision medicine strategies in drug discovery. She completed her PhD in Biomedical Informatics at Stanford University.
Sirota’s research experience in translational bioinformatics spans more than 15 years during which she has co-authored over 100 scientific publications. Her research interests lie in developing computational integrative methods and applying these approaches in the context of disease diagnostics and therapeutics with a special focus on studying the role of the immune system in disease.
The Sirota laboratory is or has been funded by NIA, NLM, NIAMS, Pfizer, March of Dimes and the Burroughs Wellcome Fund. As a young leader in the field, she was awarded the AMIA Young Investigator Award in 2017. Sirota also founded and directs the AI4ALL program at UCSF, with the goal of introducing high school girls to applications of AI and machine learning in biomedicine.
To learn more about Sirota, visit Marina Sirota on UCSF Profiles.
Related Research Recently Published by Marina Sirota
- Mortality Risk Among Patients With COVID-19 Prescribed Selective Serotonin Reuptake Inhibitor Antidepressants JAMA Network 11/15/21
- Sex-Stratified Single-Cell RNA-Seq Analysis Identifies Sex-Specific and Cell Type-Specific Transcriptional Responses in Alzheimer’s Disease Across Two Brain Regions Molecular Neurobiology 10/20/21
- Experimental and real-world evidence supporting the computational repurposing of bumetanide for APOE4-related Alzheimer’s disease Nature Aging 10/11/21
- Sex-Specific Cross Tissue Meta-Analysis Identifies Immune Dysregulation in Women With Alzheimer’s Disease Frontiers in Aging Neuroscience 9/30/21
- Transcriptomics-based drug repositioning pipeline identifies therapeutic candidates for COVID-19 Nature 6/10/21
- Decreased Mortality Rate Among COVID-19 Patients Prescribed Statins: Data From Electronic Health Records in the US Frontiers in Medicine 2/3/21
Other Sources
- Coronavirus Pandemic: Study: Some Antidepressants May Lower Risk of Death From COVID CNN News Live 11/16/21
- Experimental and real-world evidence supporting the computational repurposing of bumetanide for APOE4-related Alzheimer’s disease, Nature Aging 11/12/21
- PDF of the Sirota Nature Aging publication of 11/12/21
- Gladstone Institutes Press Release of 11/12/21 Sirota Nature Aging publication
- STAT News Review of 11/12/21 Sirota Nature Aging publication
- The maddening saga of how an Alzheimer’s ‘cabal’ thwarted progress toward a cure for decades STAT News 6/25/19