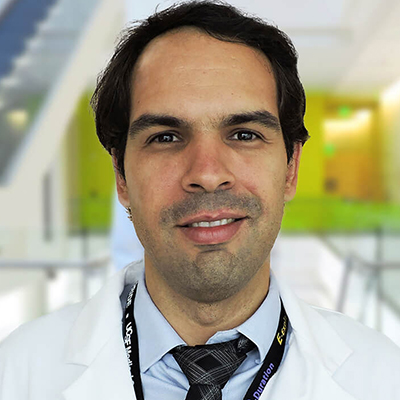
Gilmer Valdes, PhD, DABR
After earning a doctoral degree in Medical Physics from UCLA and furthering my training with a fellowship and clinical residency in Therapeutic Medical Physics at the University of Pennsylvania, I've committed myself to the development of machine learning algorithms and advanced models. These models have been instrumental in predicting patient outcomes and customizing treatments, especially for prostate and thoracic cancer patients. A significant part of my recent work involves devising methods that allow inspection and interpretation of machine learning models, as evidenced by initiatives like MediBoost , Expert Augmented Machine Learning and The Additive Tree. My proficiency in developing R and Python packages enhances the data analysis processes within cancer applications. Serving as the Director of the "Advanced Machine Learning" graduate course at UCSF, and holding joint appointments in the Radiation Oncology and Biostatistics departments, my focus has been to develop algorithms tailored to the medical field. My work has been recognized with an NIH NIBIB K08 award.
Developing interpretable and causal machine learning algorithms for their application to medicine
Specializing in Medical Physics and Therapeutic Medical Physics, my research interests lie in creating machine learning algorithms and advanced models to predict patient outcomes and individualize treatments, particularly for prostate and thoracic cancer patients. My recent work is centered on enhancing the transparency and interpretability and causality of machine learning models, alongside developing specialized R and Python packages for cancer-related data analysis. I am also committed to bringing machine learning benefits to the medical field at large.